
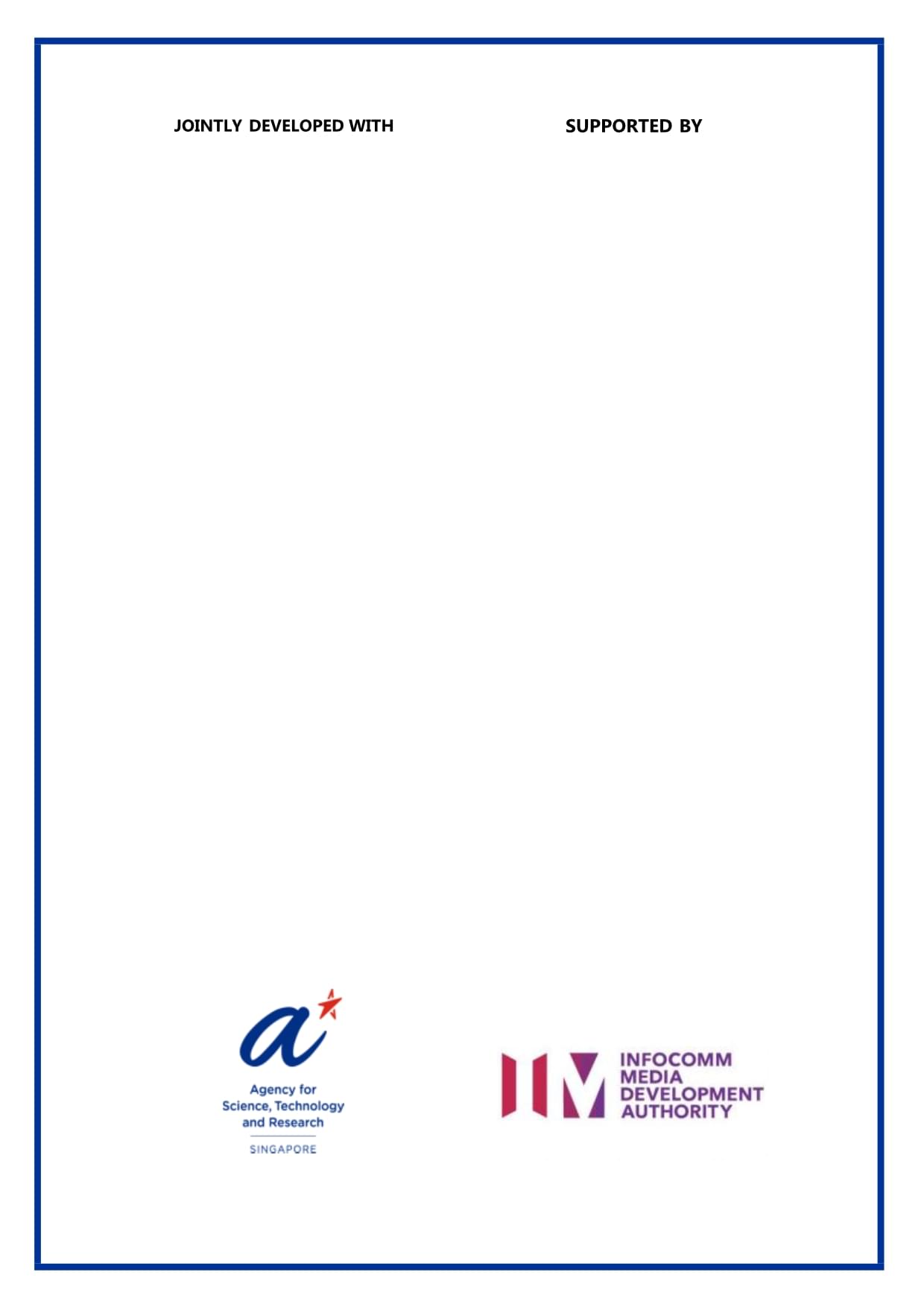



版權(quán)說(shuō)明:本文檔由用戶(hù)提供并上傳,收益歸屬內(nèi)容提供方,若內(nèi)容存在侵權(quán),請(qǐng)進(jìn)行舉報(bào)或認(rèn)領(lǐng)
文檔簡(jiǎn)介
PRIVACYENHANCINGTECHNOLOGY(PET):
PROPOSEDGUIDEONSYNTHETICDATA
GENERATION
Published15July2024VersionNumber1.0
JOINTLYDEVELOPEDWITHSUPPORTEDBY
2
TABLEOFCONTENTS
I.IntroductiontoPrivacyEnhancingTechnology(PET) 3
II.SyntheticData 4
WhatisSyntheticData? 5
UnderWhatCircumstancesisSyntheticDataUseful? 6
CaseStudies 8
III.Recommendations 10
AnnexA:HandbookonKeyConsiderationsandBestPracticesin
SyntheticDataGeneration 11
AnnexB:DataDictionaryFormat 24
AnnexC:ExamplesofMethodsofSyntheticDataGeneration 27
AnnexD:Re-identificationRisks 33
AnnexE:ExamplesofApproachesinEvaluationofRe-identification
Risks 35
ACKNOWLEDGEMENTS 41
3
I.IntroductiontoPrivacyEnhancingTechnology(PET)
PrivacyEnhancingTechnologies(PETs)areasuiteoftoolsandtechniquesthatallowtheprocessing,analysis,andextractionofinsightsfromdatawithoutrevealingtheunderlyingpersonalorcommerciallysensitivedata.ByincorporatingPETs,companiescanmaintainacompetitiveedgeinthemarketthroughleveragingtheirexistingdataassetsforinnovationwhilecomplyingwithdataprotectionregulations,reducingtheriskofdatabreachesanddemonstratingacommitmenttodataprotection.PETsarenotjustadefensivemeasure;theyareaproactivesteptowardsfosteringacultureofdataprotectionandsecuringacompany'sreputationinthedigitalage.
PETscangenerallybeclassifiedintothreekeycategorie
s1:
dataobfuscation,encrypteddataprocessing,andfederatedanalytics.PETscanalsobecombinedtoaddressvaryingneedsoforganisations.ThefollowingTable1mapsoutthecurrenttypesofPETsinthemarketandtheirkeyapplications.
Table1.TypesofPETsandtheirapplications
Categoriesof
PETs
PETs
Examplesofapplications(non-exhaustive)
Data
obfuscation
Anonymisation/pseudonymisationtechniques
?Securestorage
?Datasharingandretention
?Softwaretesting
Syntheticdatageneration
?Privacy-preservingAImachinelearning
?Datasharingandanalysis
?Softwaretesting
Differentialprivacy
?Expandingresearchopportunities
?Datasharing
Zeroknowledgeproofs
?Verifyinginformationwithout
requiringdisclosure(e.g.,ageverification)
Encrypteddataprocessing
Homomorphicencryption
?Securedatastoredincloud
1AdaptedfromOECD,“EmergingPrivacyEnhancingTechnologies:CurrentRegulatoryandPolicyApproaches,”O(jiān)ECDDigitalEconomyPapers(OECD,2023).
4
?Computingonprivate
datathatisnotdisclosed
Multi-partycomputation
(includingprivatesetintersection)
?Computingonprivate
datathatisnotdisclosed
Trustedexecutionenvironments
?Computingusing
modelsthatneedtoremainprivate
?Computingonprivate
datathatisnotdisclosed
Federatedanalytics
Federatedlearning
?Privacy-preservingAImachinelearning
Distributedanalysis
II.SyntheticData
Thisguidefocusesontheuseofsyntheticdata
2
togeneratestructureddata.Whilesyntheticdataisgenerallyfictitiousdatathatmaynotbeconsideredpersonaldataonitsown,itisnotinherentlyrisk-freeduetopossiblere-identificationrisk
s3.
Assuch,thisguideproposesgoodpracticesthatorganisationsmayadopttogeneratesyntheticdatatominimisesuchrisksforasetofcommonusecasearchetypes.Theguidealsoincludesasetofgoodpracticesandriskassessments/considerationsforgeneratingsyntheticdataaswellasgovernancecontrols,contractualprocess,andtechnicalmeasurestomitigateresidualrisks.
ThetargetaudienceforthisguideareCIOs,CTOs,CDOs,datascientists,dataprotectionpractitioners,andtechnicaldecision-makerswhomaydirectlyorindirectlybeinvolvedinthegenerationanduseofsyntheticdata.
Syntheticdataisatechnologythatisbeingactivelyresearchedanddevelopedatthetimeofpublication.Hence,thisguideisnotintendedtoprovideacomprehensiveorin-depthreviewofthetechnologyoritsassessmentmethods.Theguideisintendedtobealivingdocument,andwillbeupdatedtoensureitsrecommendationsremainrelevant.
2Therearetwotypesofsyntheticdata:fullysyntheticdataandpartiallysyntheticdata.Thisguidediscussestheuseoffullysyntheticdata.
3Inthisguide,wegenerallyrefertoprivacyrisksasre-identificationrisks.
5
WhatisSyntheticData?
Syntheticdataiscommonlyreferredtoasartificialdatathathasbeengeneratedusingapurpose-builtmathematicalmodel(includingartificialintelligence(AI)/machinelearning(ML)models)oralgorithm.Itcanbederivedbytrainingamodel(oralgorithm)onasourcedatasettomimicthecharacteristicsandstructureofthesourcedata.Goodqualitysyntheticdatacanretainthestatisticalpropertiesandpatternsofthesourcedatatoahighextent.Asaresult,performinganalysisonsyntheticdatacanproduceresultssimilartothoseyieldedwithsourcedata.
Characteristicsofsyntheticdata
Figure1showsanexampleofhowsyntheticdatamaylooklikeascomparedwiththesourcedata.Generatedsyntheticdatawillgenerallyhavedifferentdatapointsfromthesourcedata,asseenfromthetabulardata.However,thesyntheticdatawillhavestatisticalpropertiesthatareclosetothatofthesourcedata,i.e.,capturingthedistributionandstructureofthesourcedataasseenfromthetrendlinesinFigure1.
Figure1:Sourcedataversussyntheticdata
.4
Assuch,syntheticdatamaynotalwaysbeinherentlyrisk-freeasinformationaboutanindividualinthesourcedataset,orconfidentialdata,canstillbeleakedduetotheresemblanceofthesyntheticdatatothesourcedata.Therewillalsobetrade-off
s5
betweendatautilityanddataprotectionrisksinsyntheticdatageneration.However,suchriskscanbeminimisedbytakingdataprotectionintoconsiderationduringthesyntheticdatagenerationprocess.
4DiagramtakenwithmodificationfromKhaledElEmam,LucyMosquera,andRichardHoptroff,PracticalSyntheticDataGeneration(O’ReillyMedia,Inc,2020).
5Trade-offbetweendatautilityanddataprotectionrisksisfurtherdiscussedinAnnexA:Step1andStep
3inthisguide.
6
UnderWhatCircumstancesisSyntheticDataUseful?
SyntheticdatacanbeusedinavarietyofusecasesrangingfromgeneratingtrainingdatasetsforAImodelstodataanalysisandcollaboration.Theuseofsyntheticdatanotonlycanaccelerateresearch,innovation,collaboration,anddecision-makingbutalsomitigateconcernsaboutcybersecurityincidentsanddatabreaches,enablingbettercompliancewithdataprotection/privacyregulations.Table2discussesafewcommonusecasearchetypes,theirkeybenefits,andgoodpracticesthatorganisationscanfocusonwhengeneratingsyntheticdata.
Table2.Usecasearchetypesforsyntheticdata.
TypesofUseCases
KeyBenefits
GoodPracticesto
GenerateSyntheticData
Usecasearchetype1:GeneratingtrainingdatasetforAImodels
Augmenting
dataforAI/MLmodels
?Syntheticdataaddressesthechallengeoftheuserhavingtoobtainlargevolumesof
labelleddataneededfor
trainingandtestingAI/MLmodelsduetocosts,legalregulations,andproprietaryrights.
?Augmentingtrainingdatasetswithsyntheticallygeneratedlabelleddatacanbemore
cost-effective,especially
whenthesourcedatasetsaresparse.
?Addnoise*toorreducegranularityofthe
syntheticdatapoints.
?Suchfictitiousnewdatapointswillgenerallynotbeconsideredpersonaldata.
*Ifthestatistical
properties/characteristicsofthesyntheticdatais
representativeofthe
populationinquestionandnotsignificantlyskewed
towardsaspecific
individual/groupof
individualsusedassourcetrainingdata,addingof
noisemightnotbe
necessaryasre-
identificationrisksaregenerallylow.
Increasing
datadiversityforAI/ML
models
?Syntheticdatacanbeusedtosimulaterareeventsor
augmentunder-representedgroupsintrainingAImodels.
?DiversedatasetscanbeusefulinimprovingperformanceofAI/MLmodels
Usecasearchetype2:Dataanalysisandcollaboration
Datasharingandanalysis
?Underlyingtrendsorpatterns,andbiasesofthedataare
usefulfordataanalytics
regardlessofwhetherthedatasourceisrealorsynthetic.
?Balancethetrade-offs
betweendatautilityanddataprotectionby
incorporatingdataprotectionmeasures
7
?Syntheticdatacanenabledatasharingforanalysisespeciallyinindustriesandsectors,e.g.,healthcare,wherethesourcedatacanbesensitive.
throughoutthesyntheticdatagenerationprocess,forexample:
Datapreparation
?Removeoutliersfromsourcedata
?Pseudonymisesourcedata
?Employdata
minimisationand
generalisegranulardata
Syntheticdatageneration
?Addnoisebeforeoraftersyntheticdatageneration
Postsyntheticdatageneration
?Incorporatetechnical,
contractual,and
governancemeasurestomitigateanyresidualre-identificationrisks
Previewing
datafor
collaboration
?Syntheticdatacanbeusedindataexploration,analysis,andcollaborationtoprovide
stakeholderswitha
representativepreviewofthesourcedatawithoutexposingsensitiveinformation.
?Thisenablesstakeholdersto
exploreandunderstandthe
structure,relationships,and
potentialinsightswithinthe
datatogainassuranceofthedataqualitybeforefinalisinganyagreementor
collaboration.
Usecasearchetype3:Softwaretesting
System
development/software
testing
?Organisationscanuse
syntheticdatainsteadof
productiondatatofacilitatesoftwaredevelopment.
?Useofsyntheticdatacanhelporganisationsavoiddata
breachesintheeventofthedevelopmentenvironmentbeingcompromised.
?Focusongenerating
syntheticdatathat
followssemanticse.g.,format,min/maxvaluesandcategories,of
sourcedatainsteadofthestatistical
characteristicsandproperties.
RefertoAnnexAforproposedconsiderationsandgoodpracticestogeneratesyntheticdata.
8
CaseStudies
(A)TrainingAImodelforfrauddetectioninthefinancialsecto
r6
Problem:Sincethenumberoffraudulenttransactionsinthesourcedataissmallcomparedtonormal,non-fraudulenttransactions,thesourcedatadidnottrainmodelsverywellforfrauddetection.
Solution:J.P.Morgansuccessfullyusedsyntheticdataforfrauddetectionmodeltraining.AImodelswereprovidedwithsamplesofnormalandfraudulenttransactionstounderstandthetell-talesignsofsuspicioustransactions.
Benefit:Syntheticdataprovedtobemoreeffectiveintermsoftrainingmodelstodetectanomalousbehaviour.Thisisbecausethesyntheticdatausedwasdesignedtocontainahigherpercentageoffraudulenttransactions.
(B)TrainingAImodelforresearchintoAIbia
s7
Problem:Multi-labelclassificationandregressionmodelsarefrequentlyutilisedatMastercardforvariousapplications,includingfraudprevention,anti-moneylaunderingandmarketingusecasesforportfoliooptimisation.Thesemodels,whilepowerful,requirecarefulattentiontoproxiesofdemographicattributeswithintheirtrainingdata,whichcouldlearnunintendedbiases.Ensuringtheaccuracyandfairnessofthesemodelsiscomplexduetotheirmulti-labelsetting,theconfidentialityofthedemographicattributes,andthechallengesinaccessingthetrainingdatasetformodeldevelopment.
Solution:MastercardpartneredwithresearcherstodevelopnewAIbiastestingmethodsadaptedtomulti-labelsettings.Toprotecttheprivacyofthedatasharedexternally,syntheticdatawascreatedtosupportmodeltrainingandmethodologicalresearchintofairmulti-labelmodels.
Benefit:Syntheticdatawasmeasuredtobesufficientlyprivatetobesharedwithexternalresearcherswhilecapturingrealrelationshipswithinthesourcedata.Syntheticdataenablednewinsightsthatwouldnothavebeenpossiblewithouttheprivacyprotectingcharacteristicsinherenttosyntheticdata.
6J.P.Morgan,“SyntheticDataforRealInsights,”TechnologyBlog,n.d.,
/
technology/technology-blog/synthetic-data-for-real-insights
7ContributedbyMastercard
9
(C)Safeguardingpatientdatafordataanalysis
8
Problem:Priortoutilisingsyntheticdata,Johnson&Johnson(J&J)allowedexternalresearchersorconsortiatoaccesshealthcaredataforresearchproposalsvalidatedbyJ&J.Tosafeguardpatientprivacy,thedatawastransformedintoanonymisedhealthcaredata.However,feedbackreceivedindicatedthattheoverallusefulnessoftheanonymiseddata,whichreliedontraditionalanonymisationtechniques,wasnotalwayssatisfactoryanddidnotalwaysmeettherequirementsoftheresearchersorconsortia.
Solution:J&Jhasintroducedhigh-qualityAIgeneratedsyntheticdataasanadditionaloptiontoprocesstheirhealthcaredata.
Benefit:Researchersandclientshaveexperiencedsignificantlyimprovedanalysis.Whenemployedproperly,thisformofsyntheticdatacaneffectivelyrepresentthetargetpopulationandoffervariousanalyticalandscientificbenefits.
(D)Facilitatingdata
collaboration9
Problem:Apharmaceuticalcompanywantedtopurchaseheart-relatedhealthdatafromaresearchinstitutetotestoutanewhypothesis.Thehealthdata,whichwascollectedbytheresearchinstitutefromconsentingsubjects,washostedunderahighlyregulatedenvironmentasrequiredofthehealthcaresector.However,thispresentssignificantchallengesformanydataengagementactivities.
Solution:A*STARwasengagedbythepharmaceuticalcompanytobuildapipelinetocreatesyntheticcopiesoftheactualdata,whichcanthenbebroughtoutsideofthisregulatedenvironment.
Benefit:Thisallowedthepharmaceuticalcompanytopreviewthedataandbeassuredofthedataqualitypriortothehigh-valuepurchaseandaccesstotheactualdata.
8ContributedbyJohnson&Johnson(J&J)
9ContributedbyA*STAR
10
III.Recommendations
SyntheticdatahasthepotentialtodrivethegrowthofAI/MLbyenablingAImodeltrainingwhileprotectingtheunderlyingpersonaldata.ItalsoaddressesdatasetrelatedchallengesforAImodeltraining,suchasinsufficientandbiaseddata,throughenablingtheaugmentationandincreaseddiversityoftrainingdatasets.
Inaddition,syntheticdatacanbeusedtofacilitateandsupportorganisations’dataanalytics,collaborationandsoftwaredevelopmentneeds.Anaddedbenefitofusingsyntheticdatainplaceofproductiondatatofacilitatesoftwaredevelopmentisthatdatabreachescanbeavoidedintheeventthedevelopmentenvironmentiscompromised.
PDPCrecommendsasetofgoodpracticesandriskassessments/considerationsforgeneratingsyntheticdataandtoreduceanyresidualrisksfromre-identificationthroughgovernancecontrols,contractualprocess,andtechnicalmeasures(refertoAnnexA).
11
AnnexA:HandbookonKeyConsiderationsandBestPracticesinSyntheticDataGeneration
Inthishandbook,wedescribethekeyconsiderationsandbestpracticesfororganisationstoreducere-identificationrisksofsynthetictabulardatathroughafive-stepapproach.
Foranyothercomplexsyntheticdatasetsthatareunstructured,organisationsareadvisedtoconsiderhiringsyntheticdataexperts,datascientistsorindependentriskassessorstoassessandmitigatetherisksofthegeneratedsyntheticdata.
Overviewoffive-stepapproachtogeneratesyntheticdata
Step1:Knowyourdata
Beforeembarkingonanysyntheticdataproject,itisnecessarytohaveaclearunderstandingofthepurposeandusecasesofthesyntheticdataandthesourcedatathatthesyntheticdataistomimic.Thiswillhelptodeterminewhetheruseofsyntheticdatamightberelevantandidentifythepossiblerisksofusingthesyntheticdata.Someoftheconsiderationsmayinclude:
?Wheregeneraltrends/insightsofsourcedataaresensitive,organisationshouldtakenotethattheuseofsyntheticdatawillnotofferanyprotectiontothetrends/insightssincetheywillbereplicatedinthesyntheticdata.
?Wherethesyntheticdataisintendedtobereleasedpublicly,organisationsmayhavetoprioritisedataprotectionoverdatautilityinsuchcircumstances.
12
?Whererelevant,organisationsshouldalsoputinplacepropercontractualobligationsonrecipientsofsyntheticdatawherenecessarytopreventre-identificationattacksonthedata.
Withthisknowledge,themanagementanddataowner,withthehelpofrelevantstakeholderssuchasthedataanalyticsteam,shouldestablishobjectivespriortosyntheticdatagenerationtodetermineanacceptableriskthreshol
d10
ofthegeneratedsyntheticdataandtheexpectedutilityofthedata.Thiswillhelpprovideorganisationswiththeappropriatebenchmarkstoassessanytrade-offsbetweendataprotectionrisksanddatautility.
Thesebenchmarksmaybeadjustedappropriatelytomeetthebusinessobjectives,takingintoconsiderationanytrade-offsbetweendatautilityanddataprotectionrisksafterthesyntheticdatagenerationprocess,aswellassafeguardsandcontrolstomitigateorloweranyresidualrisksposedbythegeneratedsyntheticdata.Theacceptancecriteriashouldbeincorporatedintotheorganisation'sriskassessments(e.g.,enterpriseriskmanagement
framework11
ifapplicable)oraDataProtectionImpactAssessment(“DPIA”
)12.
Step2:Prepareyourdata
Whenpreparingthesource
data13
forgeneratingsyntheticdata,itisimportanttoconsiderthefollowing:
?Whatarethekeyinsightsthatneededtobepreservedinthesyntheticdata?
?Whicharethenecessarydataattributesforthesyntheticdatatomeetthebusinessobjectives?
10There-identificationriskthresholdrepresentsthelevelofre-identificationriskthatisacceptableforagivensyntheticdataset.Thereiscurrentlynouniversallyacceptednumericalvalueforriskthreshold.ForfurtherdetailsrefertoStep4(Assessre-identificationrisks).
11OrganisationsmayrefertoISO27001formoreinformationondevelopinganenterpriseriskmanagementframework.
12AnexampleofthisisPDPC’sGuidetoDataProtectionImpactAssessments.ADPIAisapplicableinthecasewherepersonaldataisinvolved.TheDPIAmaynotberelevantinsituationswherethesyntheticdatagenerationdoesnotinvolvepersonaldataprocessing.
13Thisstepassumesthatthesourcedatahasbeenproperlycleaned(suchasfixingorremovingincorrect,corrupted,incorrectlyformatted,duplicate,orincompletedata)andisofacceptablequalityforthegenerationofsyntheticdata.
13
Understandingkeyinsightstobepreserved
Toensurethatthesyntheticdatacanmeetthebusinessobjectives,organisationsneedtounderstandandidentifythetrends,keystatisticalproperties,andattribute-relationshipsinthesourcedatathatneedtobepreservedforanalysise.g.,identifyrelationshipsbetweendemographiccharacteristicsofpopulationandtheirhealthconditions.
Organisationsshouldconsider,atthispoint,whetheroutliertrendsandinsightsarenecessarytobepreservedforthebusinessobjectives.Keyconsiderationscouldincludethefollowing:
?Ifoutliersarenotnecessarytomeetthebusinessobjectivesandtheriskofre-identificationishigh,organisationsshouldconsiderremovingtheoutliers.Thiscanbedonepriortosyntheticdatagenerationoratsubsequentstagesofthesyntheticdatageneration.
?Iftheobjectiveistomimicthecharacteristicsofthesourcedataascloselyaspossible,includingoutliers,thentheorganisationmayhavetopreservetheoutliertrend/insighttomeetthebusinessobjectives.Insuchinstance,theorganisationshouldnotethatthere-identificationrisksofindividualsintheoutlierdatamaybehighandhenceputinplaceriskmitigationmeasures.
?Ifthebusinessobjectiveistobalancethenumberofdatapointsindifferentdatacategories,thenthesyntheticdatagenerationprocessitselfcanhelpmitigatetheissueofoutlierssimplybygeneratingmoreoutliers.Forexample,inadataset,thenumberofoutlierdatapointscomprisingmaleindividualsmaybebalancedwithoutlierdatapointscomprisingfemaleindividuals.
Selectingdataattributes
Basedonthekeyinsightsneeded,organisationsshouldapplydataminimisationtoextractonlytherelevantdataattributesfromthesourcedata.Thereafter,removeorpseudonymisealldirectidentifier
s14
fromtheextracteddata.
Wheregranularinformationisnotnecessary,organisationsmaygeneraliseorfurtheraddnoisetothedataatthispointoratalatersteptoreducetheriskofre-identification.Forexample,organisationscangeneraliseexactheightandweight
14RefertoPDPC’sGuidetoBasicAnonymisationonhowtoidentifydirectidentifiersinadataset.
14
informationintoheightandweightbandstoreducethepossibilityofheightandweightcombinationsbeingusedtoidentifyanyoutliers.
Organisationsshouldalsostandardiseanddocumentthedetailsoneachdataattribute(suchasdatadefinitions,standards,metricsetc.)inadatadictionary.Thisenablestheorganisationtosubsequentlyvalidatetheintegrityofthegeneratedsyntheticdatatodetectanomaliesandfixanydatainconsistencies.RefertothefollowingchecklistinTable3forkeyconsiderations.
Table3:Checklistfordatapreparation
DataPreparationChecklist
Understandkeyinsights
i.
Identifytrendsandentityrelationshipstobepreservedforsyntheticdatageneration.
ii.
Removeoutliersifsuchtrends/insightsarenotnecessary.Thiscanbeperformedpostgeneration.
Selectdataattributes
iii.
Applydataminimisationtoselectonlydataattributesthatarenecessarytomeetbusinessneeds.
iv.
Removeorpseudonymisedirectidentifiers(e.g.,name,nationalidentificationnumbers).
v.
Generalisegranulardataoraddnoise(e.g.,usingdifferentialprivac
y15)
tothe
data/modelifsuchdetailedinformationisnotnecessary.Thiscanalsobeperformedpostgeneration.
vi.
Standardiseanddocumentformat,constraints,andcategoriesofsourcedataindatadictionary(refertoAnnexBforareferencetemplate):
Format
?Standardisestringstolowerorpropercase
?Datatypes,columnnames,structures,relationships
?FrequencyofdatarecordConstraints
?Constraintsofvaluesforeachdatatype,e.g.,min-maxvalues,non-negativevalues,non-nullvalues
Category
?Typesofdatacategories
?Expectedorvalidvaluesfordataattributeswithineachdatacategory.Exampleofadatacategoryis“country”.
15Theuseofdifferentialprivacytoaddnoisetosyntheticdataiswidelydiscussedasamechanismtoreducere-identificationrisks.However,thereiscurrentlynouniversalstandardonhowtoimplementdifferentialprivacy.Moreover,thenoiseaddedmayalsoreducetheutilityofthesyntheticdata,makingitlessaccurateorusefulforcertaintypesofanalysis.
15
Step3:Generatesyntheticdata
Therearemanydifferentmethods
16
togeneratesyntheticdata,forexample,sequentialtree-basedsynthesisers,copulas,anddeepgenerativemodels(DGMs).Organisationsneedtoconsiderwhichmethodsaremostappropriate,basedontheirusecases,dataobjectives,andtypesofdata.PleaserefertoAnnexCformoreinformationonthesesyntheticdatagenerationmethods.Thereafter,organisationsmayconsidersplittingthesourcedataintotwoseparatesetse.g.,80%astrainingdataset,and20%ascontrol
dataset17
forassessingre-identificationrisksofthesyntheticdata.
Aftergeneratingsyntheticdata,itisagoodpracticefororganisationstoperformthefollowingchecksonthequalityofthegeneratedsyntheticdata:
?Dataintegrity
?Datafidelity
?Datautility
Dataintegrity
Dataintegrityensurestheaccuracy,completeness,consistency,andvalidityofthesyntheticdataascomparedwiththesourcedata.Organisationscanvalidatetheintegrityofthegeneratedsyntheticdataagainstthedictionaryofthesourcedata.
Datafidelity
Datafidelityexaminesifsyntheticdatacloselyfollowsthecharacteristicsandstatisticalattributesofthesourcedata.Thereareafewmetricsformeasuringdatafidelityandtheyaretypicallydonebystatisticallycomparingthegeneratedsyntheticdatadirectlywiththesourcedata.Organisationsshouldusetheperformancemetric(s)fordatafidelit
y18
(seeTable4)thatbestmeettheirdataobjectives.
16ThisguidemaynotbecomprehensiveincoveringallothersyntheticdatagenerationmethodssuchasBayesianmodelandvariationalautoencoders(VAE).
17RefertoApproach2inAnnexEformoredetailsontheassessmentandevaluationframeworkforquantifyingre-identificationrisk.
18ThereareothergenericmetricsdescribedhereinadditiontothoselistedinTable4.SeeKhaledElEmametal.,
“UtilityMetricsforEvaluatingSyntheticHealthDataGenerationMethods:ValidationStudy,”
JMIRMedicalInformatics10,no.4(2022)
Table4:Performancemetricsfordatafidelity
Performancemetricsgenerallyusedforassessingdatafidelity
Histogram-basedsimilarity
Measuresthesimilaritybetweensourceandsyntheticdata’sdistributionsthroughahistogramcomparisonofeachfeature.Thisensuresthesyntheticdatapreservesimportantstatisticalpropertiessuchascentraltendency(mean,median),dispersion(variance,range),anddistributionshape(skewness,kurtosis).
Correlationalsimilarity
Measuresthepreservationofrelationshipsbetweenfeaturesinthesourceandsyntheticdatasets.Forexample,ifhighereducationtypicallyleadstohigherincomeinthesourcedata,thispatternshouldalsobeevidentinsyntheticdata.
Datautility
Datautilityreferstohowwellsyntheticdatacanreplaceoraddtosourcedataforthespecificdataobjectiveoftheorganisation.
Therearedifferentapproachestoevaluatetheutilityofsyntheticdata.Thetruetestofutilityishowitperformsinreal-worldtasks.OnecommonapproachtocheckthisisbytrainingidenticalAI/MLmodelsonsyntheticandtrainingdata.T
溫馨提示
- 1. 本站所有資源如無(wú)特殊說(shuō)明,都需要本地電腦安裝OFFICE2007和PDF閱讀器。圖紙軟件為CAD,CAXA,PROE,UG,SolidWorks等.壓縮文件請(qǐng)下載最新的WinRAR軟件解壓。
- 2. 本站的文檔不包含任何第三方提供的附件圖紙等,如果需要附件,請(qǐng)聯(lián)系上傳者。文件的所有權(quán)益歸上傳用戶(hù)所有。
- 3. 本站RAR壓縮包中若帶圖紙,網(wǎng)頁(yè)內(nèi)容里面會(huì)有圖紙預(yù)覽,若沒(méi)有圖紙預(yù)覽就沒(méi)有圖紙。
- 4. 未經(jīng)權(quán)益所有人同意不得將文件中的內(nèi)容挪作商業(yè)或盈利用途。
- 5. 人人文庫(kù)網(wǎng)僅提供信息存儲(chǔ)空間,僅對(duì)用戶(hù)上傳內(nèi)容的表現(xiàn)方式做保護(hù)處理,對(duì)用戶(hù)上傳分享的文檔內(nèi)容本身不做任何修改或編輯,并不能對(duì)任何下載內(nèi)容負(fù)責(zé)。
- 6. 下載文件中如有侵權(quán)或不適當(dāng)內(nèi)容,請(qǐng)與我們聯(lián)系,我們立即糾正。
- 7. 本站不保證下載資源的準(zhǔn)確性、安全性和完整性, 同時(shí)也不承擔(dān)用戶(hù)因使用這些下載資源對(duì)自己和他人造成任何形式的傷害或損失。
最新文檔
- 小兒糖原貯積病Ⅵ型的臨床護(hù)理
- 遼寧鐵道職業(yè)技術(shù)學(xué)院《大學(xué)生職業(yè)發(fā)展與就業(yè)指導(dǎo)I》2023-2024學(xué)年第二學(xué)期期末試卷
- 銅仁學(xué)院《智慧工地》2023-2024學(xué)年第二學(xué)期期末試卷
- 深圳技術(shù)大學(xué)《射頻識(shí)別技術(shù)》2023-2024學(xué)年第二學(xué)期期末試卷
- 上海外國(guó)語(yǔ)大學(xué)附中2025年高三第二學(xué)期第一學(xué)段考試語(yǔ)文試題試卷含解析
- 江蘇省宜興市周鐵學(xué)區(qū)重點(diǎn)名校2025年招生考試(三)物理試題模擬試題含解析
- 山東省臨沂市羅莊區(qū)、河?xùn)|區(qū)、高新區(qū)三區(qū)市級(jí)名校2025年初三仿真(一)語(yǔ)文試題試卷含解析
- 泰寧縣2025屆四下數(shù)學(xué)期末復(fù)習(xí)檢測(cè)模擬試題含解析
- 臺(tái)州科技職業(yè)學(xué)院《筑牢中華民族共同體專(zhuān)題》2023-2024學(xué)年第二學(xué)期期末試卷
- 江蘇省泰州市三中學(xué)教育聯(lián)盟2024-2025學(xué)年初三下學(xué)期第一次階段考試物理試題含解析
- 學(xué)校財(cái)務(wù)人員聘任合同書(shū)
- 《健康服務(wù)與管理導(dǎo)論》期末復(fù)習(xí)筆記
- 高爾夫球場(chǎng)澆灌施工方案
- 出納員工考核試題及答案
- 河南省鄭州市2024-2025學(xué)年高三上學(xué)期1月第一次質(zhì)量預(yù)測(cè)地理試題2
- 項(xiàng)目啟動(dòng)會(huì)模板
- 2025-2030年可穿戴式睡眠監(jiān)測(cè)儀行業(yè)深度調(diào)研及發(fā)展戰(zhàn)略咨詢(xún)報(bào)告
- 《圓明園的介紹》課件
- (2025)入團(tuán)考試題庫(kù)及答案
- 掃描電子顯微鏡(SEM)-介紹-原理-結(jié)構(gòu)-應(yīng)用
- 車(chē)廂定做合同范文大全
評(píng)論
0/150
提交評(píng)論