
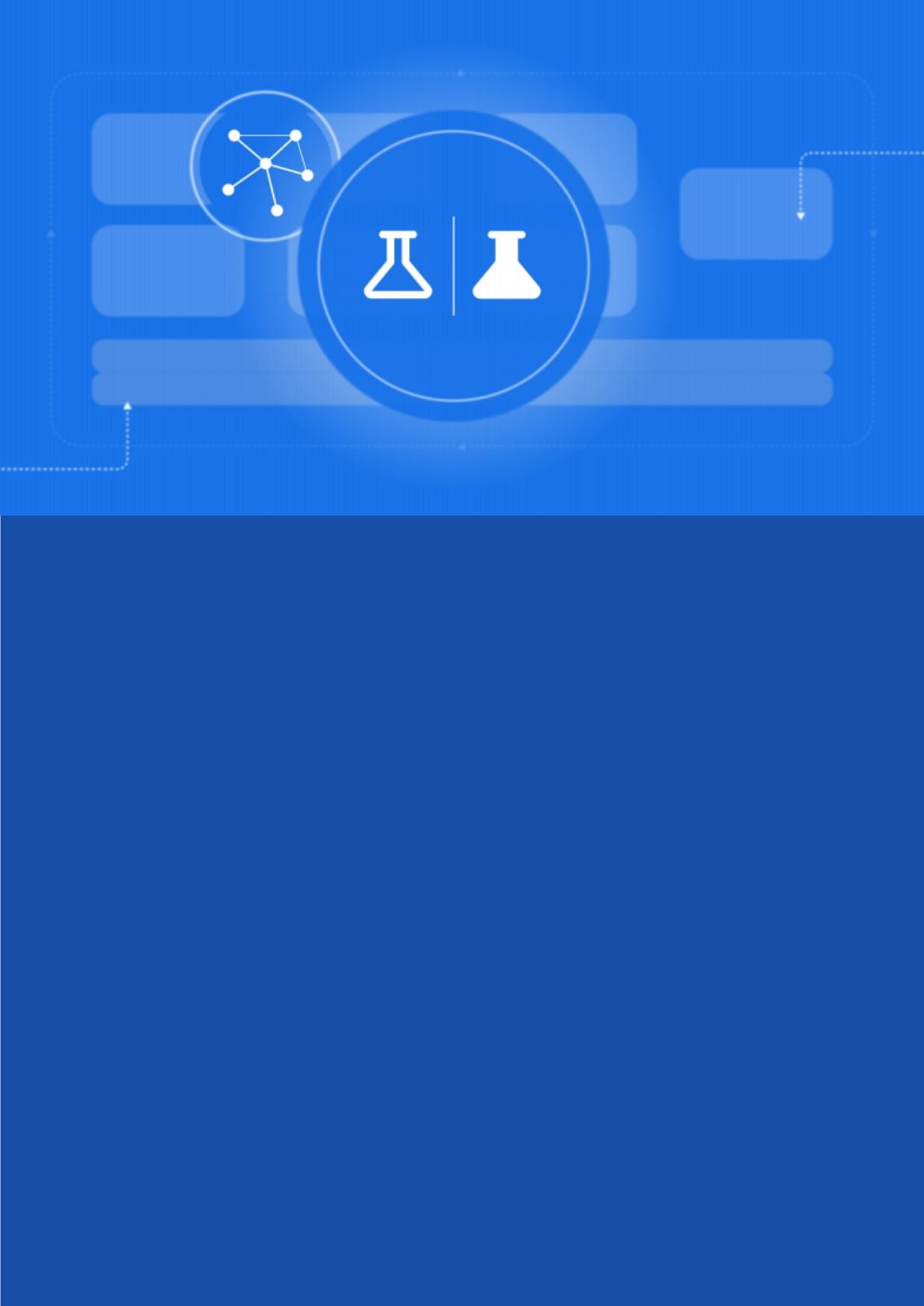



版權(quán)說(shuō)明:本文檔由用戶提供并上傳,收益歸屬內(nèi)容提供方,若內(nèi)容存在侵權(quán),請(qǐng)進(jìn)行舉報(bào)或認(rèn)領(lǐng)
文檔簡(jiǎn)介
November2024
Anew
goldenageofdiscovery
SeizingtheAIforScienceopportunity
ConorGriffin|DonWallace|JuanMateos-Garcia|HannaSchieve|PushmeetKohli
Acknowledgements
ThankyoutoLouisaBartolo,Zo?BrammerandNickSwansonforresearchsupport,andtothefollowingindividualswhosharedinsightswithusthroughinterviewsand/orfeedbackonthedraft.Allviews,andanymistakes,belongsolelytotheauthors.
?igaAvsec,NicklasLundblad,JohnJumper,MattClifford,BenSouthwood,CraigDonner,Jo?lleBarral,TomZahavy,BeenKim,SebastianNowozin,MattClancy,MatejBalog,JenniferBeroshi,NitarshanRajkumar,BrendanTracey,YannisAssael,MassimilianoCiaramita,MichaelWebb,AgnieszkaGrabska-Barwinska,
AlessandroPau,TomLue,AgataLaydon,AnnaKoivuniemi,AbhishekNagaraj,HarryLaw,TomWestgarth,GuyWard-Jackson,AriannaManzini,StefanoBianchini,SameerVelankar,AnkurVora,SébastienKrier,
JoelZLeibo,ElisaLaiH.Wong,BenJohnson,DavidOsimo,AndreaHuber,DipanjanDas,EkinDogusCubuk,JacklynnStott,KelvinGuu,KiranVodrahalli,SanilJain,TrieuTrinh,RebecaSantamaria-Fernandez,
RemiLam,VictorMartin,NeelNanda,NenadTomasev,ObumEkeke,UchechiOkereke,FrancescaPietra,RishabhAgarwal,PeterBattaglia,AnilDoshi,YianYin.
GoogleDeepMind2
Introduction
GoogleDeepMind3
Introduction
PartA:Theopportunities
PartB:Theingredients
PartC:Therisks
PartD:Thepolicyresponse
Introduction
Aquietrevolutionisbrewinginlabsaroundtheworld,wherescientists’useofAIis
growing
exponentially
.
Oneinthreepostdocs
nowuselargelanguagemodelstohelpcarryoutliterature
reviews,coding,andediting.InOctober,thecreatorsofour
AlphaFold2
system,DemisHassabisandJohnJumperbecame
Nobel
LaureatesinChemistryforusingAItopredictthestructureofproteins,alongsidethescientistDavidBaker,forhisworktodesignnewproteins.Societywillsoonstarttofeelthesebenefitsmoredirectly,with
drugs
and
materials
designedwiththehelpofAIcurrentlymakingtheirwaythroughdevelopment.
Inthisessay,wetakeatourofhowAIistransformingscientificdisciplinesfromgenomicstocomputersciencetoweatherforecasting.SomescientistsaretrainingtheirownAImodels,whileothersarefine-tuningexistingAImodels,orusingthesemodels’predictionstoacceleratetheirresearch.Scientists
areusingAIasascientificinstrumenttohelptackleimportantproblems,suchas
designingproteinsthat
bindmoretightlytodiseasetargets
,butarealsograduallytransforminghowscienceitselfispractised.
Thereisagrowingimperativebehindscientists’embraceofAI.Inrecentdecades,scientistshave
continuedtodeliverconsequentialadvances,fromCovid-19vaccinestorenewableenergy.Butit
takes
aneverlargernumberofresearcherstomakethesebreakthroughs
,andto
transformtheminto
downstreamapplications
.Asaresult,eventhoughthescientificworkforcehasgrownsignificantly
overthepasthalf-century,
risingmorethan
sevenfold
intheUSalone,thesocietalprogressthat
wewouldexpecttofollow,hasslowed.Forinstance,muchoftheworldhaswitnesseda
sustained
slowdown
inproductivitygrowththatisunderminingthequalityofpublicservices.Progresstowardsthe2030SustainableDevelopmentGoals,whichcapturethebiggestchallengesinhealth,the
environment,andbeyond,is
stalling
.
Inparticular,scientistslookingtomakebreakthroughstodayincreasinglyrunintochallengesrelatingtoscaleandcomplexity,fromtheever-growingliteraturebasetheyneedtomaster,totheincreasinglycomplexexperimentstheywanttorun.
M
oderndeeplearningmethods
areparticularlywell-suited
tothese
scaleandcomplexitychallenges
andcancompressthetimethatfuturescientificprogresswouldotherwiserequire.Forinstance,instructuralbiology,asinglex-raycrystallographyexperimenttodeterminethestructureofaprotein
cantakeyearsofworkandcostapproximately$100,000
,
dependingontheprotein.The
AlphaFoldProteinStructureDatabase
nowprovidesinstantaccessto200millionpredictedproteinstructuresforfree.
ThepotentialbenefitsofAItosciencearenotguaranteed.AsignificantshareofscientistsalreadyuseLLM-basedtoolstoassistwitheverydaytasks,suchascodingandediting,buttheshareofscientistsusingAI-centricresearchapproaches
ismuchlower,albeit
risingrapidly
.IntherushtouseAI,some
earlyscientificusecaseshavehad
questionableimpact
.PolicymakerscanhelpaccelerateAI’suseandsteerittowardshigher-impactareas.The
USDepartmentofEnergy,
the
EuropeanCommission
,theUK’s
Royal
Society,
andthe
USNationalAcademies
,amongothers,haverecentlyrecognisedtheAIforScienceopportunity.Butnocountryhasyetputacomprehensivestrategyinplacetoenableit.
GoogleDeepMind4
Introduction
PartA:Theopportunities
PartB:Theingredients
PartC:Therisks
PartD:Thepolicyresponse
Wehopeouressaycaninformsuchastrategy.Itisaimedatthosewhomakeandinfluencesciencepolicy,andfundingdecisions.Wefirstidentify5opportunitieswherethereisagrowingimperativetouseAIinscienceandexaminetheprimaryingredientsneededtomakebreakthroughsinthese
areas.Wethenexplorethemostcommonly-citedrisksfromusingAIinscience,suchastoscientificcreativityandreliability,andarguethatAIcanultimatelybenetbeneficialineacharea.WeconcludewithfourpublicpolicyideastohelpusherinanewgoldenageofAI-enabledscience.
ThroughouttheessaywedrawoninsightsfromovertwodozeninterviewswithexpertsfromourownAIforScienceprojects,aswellasexternalexperts.Theessaynaturallyreflectsourvantagepointasaprivatesectorlab,butwebelievethecasewemakeisrelevantforthewholeofscience.WehopethatreaderswillrespondbysharingtheirtakeonthemostimportantAIforScienceopportunities,
ingredients,risksandpolicyideas.
PartA:Theopportunities06
PartB:Theingredients13
PartC:Therisks25
PartD:Thepolicyresponse32
GoogleDeepMinds
PartA
The
opportunities
6
Introduction
PartA:Theopportunities
PartB:Theingredients
PartC:Therisks
PartD:Thepolicyresponse
Theopportunities
Scientistsaimtounderstand,predict,andinfluencehowthenaturalandsocialworldswork,toinspireandsatisfycuriosity,andtotackleimportantproblemsfacingsociety.
Technologiesandmethods
,
likethemicroscope,x-raydiffraction,andstatistics,arebothproductsofscienceandenablersof
it.Overthepastcentury,scientistshaveincreasinglyreliedontheseinstrumentstocarryouttheir
experimentsandadvancetheirtheories.Computationaltoolsandlarge-scaledataanalysishave
becomeparticularlyimportant,enablingeverythingfromthediscoveryoftheHiggsbosontothe
mappingofthehumangenome.Fromoneview,scientists’growinguseofAIisalogicalextensionofthislong-runningtrend.Butitmayalsosignalsomethingmuchmoreprofound-adiscontinuousleapinthelimitsofwhatscienceiscapableof.
RatherthanlistingallareaswhereitispossibletouseAI,wehighlightfiveopportunitieswherewethinkthereisanimperativetouseit.Theseopportunitiesapplyacrossdisciplinesandaddressaspecific
bottleneck,relatedtoscaleandcomplexity,thatscientistsincreasinglyfaceatdifferentpointsinthescientificprocess,fromgeneratingpowerfulnovelhypothesestosharingtheirworkwiththeworld.
5opportunitiestoacceleratesciencewithAl
1.Knowledge2.Data3.Experiments
TransformhowscientistsdigestGenerate,extract,andannotateSimulate,accelerateandinform
andcommunicateknowledgelargescientificdatasetscomplexexperiments
Q
4.Models5.Solutions
ModelcomplexsystemsandhowIdentifynovelsolutionstoproblems
theircomponentsinteractwithlargesearchspaces
GoogleDeepMind7
Introduction
PartA:Theopportunities
PartB:Theingredients
PartC:Therisks
PartD:Thepolicyresponse
1.Knowledge
Transformhowscientistsdigestandcommunicateknowledge
Tomakenewdiscoveries,scientistsneedtomasterapre-existingbodyofknowledgethatcontinuestogrow
exponentially
andbecomeevermorespecialised.This
‘burdenofknowledge
’helpsexplainwhyscientists
making
transformative
discoveries
areincreasinglyolder,interdisciplinary,andlocatedateliteuniversities,andwhythe
shareofpapers
authoredbyindividuals,orsmallteams,isdeclining,eventhough
smallteamsareoftenbetter-placedtoadvancedisruptivescientificideas
.Whenit
comestosharingtheirresearchtherehavebeenwelcomeinnovationssuchaspreprintserversandcoderepositories,butmostscientistsstillsharetheirfindingsin
dense,jargon-heavy,
English-only
papers
.Thiscanimpederatherthanigniteinterestinscientists’work,includingfrompolicymakers,businesses,andthepublic.
ScientistsarealreadyusingLLMs,andearlyscientificassistantsbasedonLLMs,tohelpaddress
thesechallenges,suchasby
synthesisingthemostrelevantinsights
fromtheliterature.Inanearly
demonstration
,ourScienceteam
usedour
Gemini
LLMtofind,extract,andpopulatespecificdatafromthemostrelevantsubsetof200,000papers,withinaday.Upcominginnovations,suchasfine-tuningLLMsonmorescientificdataandadvancesinlongcontextwindowsandcitationuse,will
steadilyimprovethesecapabilities.Asweexpandonbelow,theseopportunitiesarenotwithoutrisk.Buttheyprovideawindowtofundamentallyrethinkcertainscientifictasks,suchaswhatitmeansto‘read’or‘write’ascientificpaperinaworldwhereascientistcanuseanLLMtohelpcritiqueit,tailoritsimplicationsfordifferentaudiences,ortransformitintoan‘interactivepaper’or
audioguide
.
2.Data
Generate,extract,andannotatelargescientificdatasets
Despitepopularnarrativesaboutaneraofdataabundance,thereisachroniclackofscientificdataonmostofthenaturalandsocialworld,fromthesoil,deepoceanandatmosphere,totheinformaleconomy.AIcouldhelpindifferentways.Itcouldmakeexistingdatacollectionmoreaccurate,forexampleby
reducingthenoiseanderrorsthatcanoccurwhen
sequencingDNA
,
detectingcelltypesinasample,
or
capturinganimalsounds
.ScientistscanalsoexploitLLMs’growingabilitytooperateacrossimages,videoandaudio,toextracttheunstructuredscientificdatathatisburiedinscientificpublications,
archives,andlessobviousresourcessuchasinstructionalvideos,andconvertitintostructureddatasets.
AIcanalsohelptoannotatescientificdatawiththesupportinginformationthatscientistsneedinordertouseit.Forexample,atleastone-thirdofmicrobialproteins
arenotreliablyannotated
withdetailsaboutthefunction(s)thattheyarethoughttoperform.In2022,ourresearchers
usedAIto
predictthefunctionofproteins
,leadingtonewentriesinthe
UniProt
,
Pfam
and
InterPro
databases.
GoogleDeepMind8
Introduction
PartA:Theopportunities
PartB:Theingredients
PartC:Therisks
PartD:Thepolicyresponse
AImodels,oncevalidated,canalsoserveasnewsourcesofsyntheticscientificdata.Forexample,our
AlphaProteo
proteindesignmodelistrainedonmorethan100millionAI-generatedprotein
structuresfromAlphaFold2,alongwithexperimentalstructuresfromthe
ProteinDataBank
.TheseAIopportunitiescancomplementandincreasethereturnonothermuch-neededeffortstogeneratescientificdata,suchas
digitisingarchives
,orfundingnewdatacapturetechnologiesandmethods,likeeffortsunderwayinsinglecellgenomicstocreatepowerfuldatasetsofindividualcellsin
unprecedenteddetail.
3.Experiments
Simulate,accelerateandinformcomplexexperiments
Manyscientificexperimentsareexpensive,complex,andslow.Somedonothappenatallbecauseresearcherscannotaccessthefacilities,participantsorinputsthattheyneed.Fusionisacase
inpoint.Itpromisesanenergysourcethatispracticallylimitless,emission-freeandcouldenable
thescalingofenergy-intensiveinnovations,likedesalination.Torealisefusion,scientistsneedto
createandcontrolplasma-afourthfundamentalstateofmatter.However,thefacilitiesneededarehugelycomplextobuild.
ITER
’sprototypetokamakreactorbeganconstructionin2013,butplasmaexperimentsare
notsettobegin
untilthemid-2030sattheearliest,althoughothershopetobuildsmallerreactorsonshortertimelines.
AIcouldhelptosimulatefusionexperimentsandenablemuchmoreefficientuseofsubsequentexperimenttime.Oneapproachistorunreinforcementlearningagentsonsimulationsofphysicalsystems.Between2019and2021,ourresearcherspartneredwiththeSwissFederalInstituteof
TechnologyLausanneto
demonstrate
howtouseRLtocontroltheshapeofplasmainasimulationofatokamakreactor.Theseapproachescouldbeextendedtootherexperimentalfacilities,suchas
particleaccelerators
,
telescopearrays
,or
gravitationalwavedetectors
.
UsingAItosimulateexperimentswilllookverydifferentacrossdisciplines,butacommonthreadis
thatthesimulationswillofteninformandguidephysicalexperiments,ratherthansubstituteforthem.Forexample,theaverageperson
hasmorethan9,000
missensevariants,orsinglelettersubstitutionsintheirDNA.Mostofthesegeneticvariantsarebenignbutsomecandisruptthefunctionsthat
proteinsperform,contributingtoraregeneticdiseaseslikecysticfibrosisaswellascommondiseaseslikecancer.Physicalexperimentstotesttheeffectsofthesevariantsareoftenlimitedtoasingle
protein.Our
AlphaMissensemodel
classifies89%ofthe71millionpotentialhumanmissensevariants
aslikelyharmfulorbenign,enablingscientiststofocustheirphysicalexperimentsonthemostlikelycontributorstodisease.
GoogleDeepMind9
Introduction
PartA:Theopportunities
PartB:Theingredients
PartC:Therisks
PartD:Thepolicyresponse
Allpossible71millionhumanmissensevariants
AlphaMissensepredictions:
Likelybenign57%
Likelypathogenic
32%
.Uncertain
11%
Humanannotations:
Seeninhumans~6%
Confirmedbyhumanexperts~0.1%
AlphaMissensepredictedthepathogenicityofallpossible71millionmissensevariants.Itclassified89%
-predicting57%werelikelybenignand32%werelikelypathogenic.
4.Models
Modelcomplexsystemsandhowtheircomponentsinteract
Ina
1960paper,
theNobelPrizewinningphysicistEugeneWignermarvelledatthe“unreasonable
effectiveness”ofmathematicalequationsformodellingimportantnaturalphenomena,suchas
planetarymotion.However,overthepasthalfcentury,modelsthatrelyonsetsofequationsor
otherdeterministicassumptionshavestruggledtocapture
thefullcomplexityofsystemsinbiology,
economics,weather,andelsewhere
.Thisreflectsthesheernumberofinteractingpartsthatmakeupthesesystems,aswellastheirdynamismandpotentialforemergent,randomorchaoticbehaviour.Thechallengesinmodellingthesesystemsimpedesscientists’abilitytopredictorcontrolhowtheywillbehave,includingduringshocksorinterventions,suchasrisingtemperatures,anewdrug,ortheintroductionofataxchange.
GoogleDeepMind10
Introduction
PartA:Theopportunities
PartB:Theingredients
PartC:Therisks
PartD:Thepolicyresponse
AIcouldmoreaccuratelymodelthesecomplexsystemsbyingestingmoredataaboutthem,andlearningmorepowerfulpatternsandregularitieswithinthisdata.Forexample,modernweather
forecastingisatriumphofscienceandengineering.Forgovernmentsandindustry,itinforms
everythingfromrenewableenergyplanningtopreparingfor
hurricanes
and
floods
.Forthepublic,
theweatheristhemostpopularnon-brandedqueryonGoogleSearch.Traditional
numeralprediction
methods
arebasedoncarefully-definedphysicsequationsthatprovideaveryuseful,yetimperfect,approximationoftheatmosphere’scomplexdynamics.Theyarealsocomputationallyexpensiveto
run.In2023,wereleaseda
deeplearningsystem
thatpredictsweatherconditionsupto10daysinadvance,whichoutperformedtraditionalmodelsonaccuracyandpredictionspeed.Asweexpandonbelow,usingAItoforecastweathervariablescouldalsohelptomitigateandrespondtoclimatechange.Forinstance,whenpilotsflythroughhumidregionsitcancausecondensationtrailsthat
contributeto
aviation’sglobalwarmingimpact.Googlescientists
recentlyused
AItopredictwhenandwherehumidregionsmayarisetohelppilotsavoidflyingthroughthem.
Inmanycases,AIwillenrichtraditionalapproachestomodellingcomplexsystemsratherthanreplacethem.Forexample,agent-basedmodellingsimulatesinteractionsbetweenindividualactors,like
firmsandconsumers,tounderstandhowtheseinteractionsmightaffectalargermorecomplex
systemliketheeconomy.Traditionalapproachesrequirescientiststospecifybeforehandhowthesecomputationalagentsshouldbehave.Ourresearchteams
recentlyoutlined
howscientistscoulduseLLMstocreatemoreflexiblegenerativeagentsthatcommunicateandtakeactions,suchassearchingforinformationormakingpurchases,whilealsoreasoningaboutandrememberingtheseactions.
Scientistscouldalsousereinforcementlearningtostudyhowtheseagentslearnandadapttheirbehaviourin
moredynamicsimulations
,forexampleinresponsetotheintroductionofnewenergypricesorpandemicresponsepolicies.
5.Solutions
Identifynovelsolutionstoproblemswithlargesearchspaces
Manyimportantscientificproblemscomewithapracticallyincomprehensiblenumberofpotential
solutions.Forexample,biologistsandchemistsaimtodeterminethestructure,characteristics,andfunction(s)ofmoleculessuchasproteins.Onegoalofsuchworkistohelpdesignnovelversionsofthesemoleculestoserveasantibodydrugs,plastic-degradingenzymesornewmaterials.However,todesignasmallmoleculedrug,scientistsface
morethan10
60
potentialoptions.Todesignaproteinwith400standardaminoacids,theyface20400options.Theselargesearchspacesarenotlimitedtomoleculesbutarecommonplaceformanyscientificproblems,suchasfindingthebestprooffora
mathsproblem,themostefficientalgorithmforacomputersciencetask,orthe
bestarchitecturefor
acomputerchip
.
GoogleDeepMind11
Traditionally,scientistsrelyonsomecombinationofintuition,trialanderror,iteration,orbruteforce
computingtofindthebestmolecule,proof,oralgorithm.However,thesemethodsstruggletoexploitthehugespaceofpotentialsolutions,leavingbetteronesundiscovered.AIcan
openupnewpartsof
thesesearchspaces
whilealsohominginmorequicklyonthesolutionsthataremostlikelytobeviableanduseful-adelicatebalancingact.Forexample,inJuly,our
AlphaProofandAlphaGeometry2
systemscorrectlysolvedfouroutofsixproblemsfromthe
InternationalMathematicalOlympiad
,
anelitehighschoolcompetition.ThesystemsmakeuseofourGeminiLLMarchitecturetogeneratealargenumberofnovelideasandpotentialsolutionsforagivenmathsproblem,andcombinethiswithsystemsgroundedinmathematicallogicthatcaniterativelyworktowardsthecandidatesolutionsthataremostlikelytobecorrect.
AIscientistsorAI-empoweredscientists?
ThisgrowinguseofAIinscience,andtheemergenceofearlyAIscientificassistants,raisesquestionsabouthowfastandhowfarthecapabilitiesofAImayadvanceandwhatthiswillmeanforhuman
scientists.CurrentLLM-basedAIscientificassistantsmakearelativelysmallcontributiontoa
relativelynarrowrangeoftasks,suchassupportingliteraturereviews.Thereareplausiblenear-termscenariosinwhichtheybecomebetteratthesetasksandbecomecapableofmoreimpactfulones,suchashelpingtogeneratepowerfulhypotheses,orhelpingtopredicttheoutcomesofexperiments.
However,currentsystemsstillstrugglewiththedeepercreativityand
reasoning
thathumanscientistsrelyonforsuchtasks.
EffortsareunderwaytoimprovetheseAIcapabilities
,forexamplebycombiningLLMswithlogicaldeductionengines,asinour
AlphaProofandAlphaGeometry2
examples,butfurtherbreakthroughsareneeded.Theabilitytoaccelerateorautomateexperimentswillalsobeharderforthosethatrequirecomplicatedactionsinwetlabs,interactingwithhumanparticipants,orlengthy
processes,suchasmonitoringdiseaseprogression.Althoughagain,workisunderwayinsomeoftheseareas,suchasnewtypesoflaboratoryroboticsand
automatedlabs
.
EvenasAIsystems’capabilitiesimprove,thegreatestmarginalbenefitwillcomefromdeploying
theminusecasesthatplaytotheirrelativestrengths-suchastheabilitytorapidlyextractinformationfromhugedatasets-andwhichhelpaddressgenuinebottleneckstoscientificprogresssuchasthefiveopportunitiesoutlinedabove,ratherthanautomatingtasksthathumanscientistsalreadydowell.AsAIenablescheaperandmorepowerfulscience,demandforscienceandscientistswillalsogrow.
Forexample,recentbreakthroughshavealreadyledtoaslewofnewstartupsinareaslike
protein
design
,
materialscience
and
weatherforecasting
.Unlikeothersectors,anddespite
pastclaimstothe
contrary,
futuredemandforscienceappearspracticallylimitless.Newadvances
havealwaysopened
upnew,unpredictableregions
inthescientificmapofknowledge,andAIwilldosimilar.As
envisioned
byHerbertSimon,AIsystemswillalsobecomeobjectsofscienceresearch,withscientistssettoplayaleadingroleinevaluatingandexplainingtheirscientificcapabilities,aswellasindevelopingnew
typesofhuman-AIscientificsystems.
GoogleDeepMind12
PartB
The
ingredients
13
Introduction
PartA:Theopportunities
PartB:Theingredients
PartC:Therisks
PartD:Thepolicyresponse
Theingredients
WeareinterestedintheingredientsthatambitiousAIforScienceeffortsneedtosucceed-bothat
theindividualresearcheffortlevelandatthelevelofthescienceecosystem,wherepolicymakershavemorescopetoshapethem.Theexpertsthatweinterviewedroutinelycitedseveralingredientsthat
weorganisedintoatoymodel,whichwecalltheAIforScienceproductionfunction.Thisproductionfunctionisnotmeanttobeexhaustive,prescriptive,oraneatlinearprocess.Theingredientswillbeintuitivetomany,butourinterviewsrevealedanumberoflessonsaboutwhattheylooklikeinpracticewhichwesharebelow.
TheAIforscienceproductionfunction
Startingpoint
InfrastructureDoingtheresearch
Drivingimpact
Adoption
Partnerships
Safety&responsibility
Organisationaldesign
Problemselection
Interdisciplinarity
Evaluations
Compute
Data
GoogleDeepMind14
Introduction
PartA:Theopportunities
PartB:Theingredients
PartC:Therisks
PartD:Thepolicyresponse
1.Problemselection
Pursueambitious,AI-shapedproblems
Scientificprogressrestsonbeingabletoidentifyanimportantproblemandasktherightquestion
abouthowtosolveit.In
theirexploration
intothegenesisofscientificbreakthroughs,Venkatesh
NarayanamurtiandJeffreyY.Tsaodocumenthowimportantthereciprocalandrecursiverelationshipbetweenquestionsandanswersis,includingtheimportanceofaskingambitiousnewquestions.
OurScienceteamstartsbythinkingaboutwhetherapotentialresearchproblemissignificantenoughtojustifyasubstantialinvestmentoftimeandresources.OurCEODemisHassabishasamentalmodeltoguidethisassessment:thinkingaboutallofscienceasatreeofknowledge.Weareparticularly
interestedintheroots-fundamental‘rootnodeproblems’like
proteinstructureprediction
or
quantumchemistry
that,ifsolved,couldunlockentirelynewbranchesofresearchandapplications.
ToassesswhetherAIwillbesuitableandadditive,welookforproblemswithcertaincharacteristics,
suchashugecombinatorialsearchspaces,largeamountsofdata,andaclearobjectivefunctionto
benchmarkperformanceagainst.OftenaproblemissuitableforAIinprinciple,buttheinputsaren’t
yetinplaceanditneedstobestoredforlater.Oneoftheoriginal
inspirations
forAlphaFoldwas
conversationsthatDemishadmanyyearspriorasastudentwithafriendwhowasobsessedwith
theproteinfoldingproblem.Manyrecentbreakthroughsalsofeaturethiscomingtogetherofan
importantscientificproblemandanAIapproachthathasreachedapointofmaturity.Forexample,
our
fusion
effortwasaidedbyanovel
reinforcementlearningalgorithm
calledmaximumaposteriori
policyoptimization,whichhadonlyjustbeenreleas
溫馨提示
- 1. 本站所有資源如無(wú)特殊說(shuō)明,都需要本地電腦安裝OFFICE2007和PDF閱讀器。圖紙軟件為CAD,CAXA,PROE,UG,SolidWorks等.壓縮文件請(qǐng)下載最新的WinRAR軟件解壓。
- 2. 本站的文檔不包含任何第三方提供的附件圖紙等,如果需要附件,請(qǐng)聯(lián)系上傳者。文件的所有權(quán)益歸上傳用戶所有。
- 3. 本站RAR壓縮包中若帶圖紙,網(wǎng)頁(yè)內(nèi)容里面會(huì)有圖紙預(yù)覽,若沒(méi)有圖紙預(yù)覽就沒(méi)有圖紙。
- 4. 未經(jīng)權(quán)益所有人同意不得將文件中的內(nèi)容挪作商業(yè)或盈利用途。
- 5. 人人文庫(kù)網(wǎng)僅提供信息存儲(chǔ)空間,僅對(duì)用戶上傳內(nèi)容的表現(xiàn)方式做保護(hù)處理,對(duì)用戶上傳分享的文檔內(nèi)容本身不做任何修改或編輯,并不能對(duì)任何下載內(nèi)容負(fù)責(zé)。
- 6. 下載文件中如有侵權(quán)或不適當(dāng)內(nèi)容,請(qǐng)與我們聯(lián)系,我們立即糾正。
- 7. 本站不保證下載資源的準(zhǔn)確性、安全性和完整性, 同時(shí)也不承擔(dān)用戶因使用這些下載資源對(duì)自己和他人造成任何形式的傷害或損失。
最新文檔
- 2025年度環(huán)保產(chǎn)業(yè)園區(qū)規(guī)劃設(shè)計(jì)咨詢合同
- 貴州2024年貴州省重點(diǎn)產(chǎn)業(yè)人才蓄水池崗位專(zhuān)項(xiàng)簡(jiǎn)化程序招聘17人筆試歷年參考題庫(kù)附帶答案詳解
- 衡陽(yáng)2025年湖南衡陽(yáng)市市直衛(wèi)健系統(tǒng)人才引進(jìn)177人筆試歷年參考題庫(kù)附帶答案詳解
- 鹽城江蘇鹽城市教育局招錄政府購(gòu)買(mǎi)服務(wù)用工人員筆試歷年參考題庫(kù)附帶答案詳解
- 梧州2025年廣西梧州市公安局招聘輔警274人筆試歷年參考題庫(kù)附帶答案詳解
- 2025年中國(guó)天然生漆市場(chǎng)調(diào)查研究報(bào)告
- 2025年中國(guó)內(nèi)飾件市場(chǎng)調(diào)查研究報(bào)告
- 2025至2031年中國(guó)高光澤丙烯酸外墻涂料行業(yè)投資前景及策略咨詢研究報(bào)告
- 2025年舞廳效果燈項(xiàng)目可行性研究報(bào)告
- 2025至2031年中國(guó)羽絨衫行業(yè)投資前景及策略咨詢研究報(bào)告
- 低壓電工考試題庫(kù)(含答案)
- 邊坡抗滑樁計(jì)算
- 【新版本】華為 H12-711 V4.0 HCIA-Security 認(rèn)證華為安全題庫(kù)(含答案)
- 村衛(wèi)生室2023年度績(jī)效考核評(píng)分細(xì)則(基本公共衛(wèi)生服務(wù))
- 關(guān)聯(lián)公司合作合同
- 2022人臉識(shí)別安全白皮書(shū)
- 【建模教程】-地質(zhì)統(tǒng)計(jì)學(xué)礦體建模簡(jiǎn)明教材
- DB23T 2656-2020樺樹(shù)液采集技術(shù)規(guī)程
- 重源煤礦 礦業(yè)權(quán)價(jià)款計(jì)算書(shū)
- PSM工藝安全管理
- GB/T 21872-2008鑄造自硬呋喃樹(shù)脂用磺酸固化劑
評(píng)論
0/150
提交評(píng)論